Neural Network as an Universal Approximator
Contents
11. Neural Network as an Universal Approximator¶
import numpy as np
import torch
import torch.utils.data as Data
import torch.nn as nn
import torch.nn.functional as F
import torch.optim as optim
from torch.autograd import Variable
from torchvision import datasets, transforms
from torchvision.utils import make_grid , save_image
%matplotlib inline
from sklearn.preprocessing import StandardScaler
import matplotlib.pyplot as plt
architecture_name = "regularized_autoencoder"
ngpu=1
device = torch.device("cuda:0" if (torch.cuda.is_available() and ngpu > 0) else "cpu")
device
device(type='cuda', index=0)
x1 = 2*np.pi*np.linspace(0,1.5,10000)
x2 = x1*np.cos(2*x1)
x3 = np.exp(-x1)*x1
X_data = np.concatenate((x1.reshape(x1.shape[0],1),\
x2.reshape(x1.shape[0],1)),axis=1)
X_data = StandardScaler().fit_transform(X_data)
X = torch.Tensor(X_data[:,0]).type(torch.FloatTensor)
y = torch.Tensor(X_data[:,1]).type(torch.FloatTensor)
# X_tc = torch.Tensor(X_data).type(torch.FloatTensor)
# print(X_tc.shape, X_tc.type())
# torch.Size([1000, 3]) torch.FloatTensor
plt.plot(x1,x2)
[<matplotlib.lines.Line2D at 0x7f71e100bd90>]
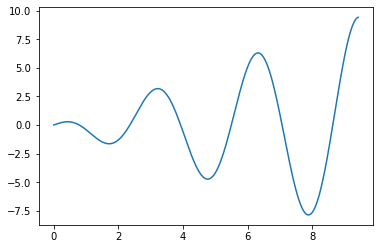
def create_loader(X,y,batch_size=50,workers=12):
torch_dataset = Data.TensorDataset(X,y)
loader = Data.DataLoader(
dataset = torch_dataset,
batch_size = batch_size,
shuffle=True)
return loader
11.1. Usando weight_decay do Adam¶
class Net(nn.Module):
def __init__(self,h_dim=1,ngpu=ngpu):
super(Net, self).__init__()
self.h_dim = h_dim
self.hidden = nn.Linear(1, self.h_dim)
self.act1 = nn.ReLU()
self.out = nn.Linear(self.h_dim, 1)
def forward(self, x):
h = self.act1(self.hidden(x))
return self.out(h)
def init_weights(m):
if type(m) == nn.Linear:
torch.nn.init.xavier_normal_(m.weight).to(device)
m.bias.data.fill_(0.001)
def define_model(model_class,h_dim):
# Instanciamos as redes
net = model_class(h_dim).to(device)
if (device.type == 'cuda') and (ngpu > 1):
net = nn.DataParallel(net, list(range(ngpu)))
# Inicializamos os pesos
net.apply(init_weights)
learning_rate, beta1, beta2 = [1e-3,0.9,0.999]
eps,weight_decay,amsgrad = [1e-12,0.0,False]
net_optimizer = optim.Adam(net.parameters(),
lr=learning_rate,
betas=(beta1,beta2),
eps=eps,
weight_decay=weight_decay, # regularization: https://pytorch.org/docs/stable/_modules/torch/optim/adam.html#Adam
amsgrad=amsgrad)
return net,net_optimizer
mse_loss = nn.MSELoss()
def test_approximator(net,X,y,h_dim):
y_pred = net(X.view(-1,1).to(device)).detach()
plt.plot(X.cpu().numpy(),y.cpu().numpy(),label="real")
plt.plot(X.cpu().numpy(),y_pred.cpu().numpy(),label="approx")
plt.xlabel(f"{h_dim}")
plt.legend(loc=0)
plt.show()
loader = create_loader(X,y,batch_size=5,workers=12)
# net,net_optimizer = define_model(Net,5)
models = [define_model(Net,h_dim) for h_dim in [1,5,20,30,50,100]]
def train_model(model,optimizer):
for epoch in range(20):
loss_ = []
for _, (X_train,y_train) in enumerate(loader):
X_train = X_train.view(-1,1).to(device)
y_train = y_train.view(-1,1).to(device)
y_pred = model(X_train)
loss = mse_loss(y_pred,y_train)
loss.backward()
optimizer.step()
optimizer.zero_grad()
# print("Training loss for {} epoch: {}".format(epoch, loss))
return model
model_1 = train_model(models[0][0],models[0][1])
model_5 = train_model(models[1][0],models[1][1])
model_20 = train_model(models[2][0],models[2][1])
model_30 = train_model(models[3][0],models[3][1])
model_50 = train_model(models[4][0],models[4][1])
model_100 = train_model(models[5][0],models[5][1])
y_pred = []
y_pred.append(model_1(X.view(-1,1).to(device)).detach())
y_pred.append(model_5(X.view(-1,1).to(device)).detach())
y_pred.append(model_20(X.view(-1,1).to(device)).detach())
y_pred.append(model_30(X.view(-1,1).to(device)).detach())
y_pred.append(model_50(X.view(-1,1).to(device)).detach())
y_pred.append(model_100(X.view(-1,1).to(device)).detach())
plt.figure(figsize=(20,15))
plt.subplot(231)
plt.plot(X.cpu().numpy(),y.cpu().numpy(),label="real")
plt.plot(X.cpu().numpy(),y_pred[0].detach().cpu().numpy(),label="model_1")
plt.legend(loc=0)
plt.subplot(232)
plt.plot(X.cpu().numpy(),y.cpu().numpy(),label="real")
plt.plot(X.cpu().numpy(),y_pred[1].detach().cpu().numpy(),label="model_5")
plt.legend(loc=0)
plt.subplot(233)
plt.plot(X.cpu().numpy(),y.cpu().numpy(),label="real")
plt.plot(X.cpu().numpy(),y_pred[2].detach().cpu().numpy(),label="model_20")
plt.legend(loc=0)
plt.subplot(234)
plt.plot(X.cpu().numpy(),y.cpu().numpy(),label="real")
plt.plot(X.cpu().numpy(),y_pred[3].detach().cpu().numpy(),label="model_30")
plt.legend(loc=0)
plt.subplot(235)
plt.plot(X.cpu().numpy(),y.cpu().numpy(),label="real")
plt.plot(X.cpu().numpy(),y_pred[4].detach().cpu().numpy(),label="model_50")
plt.legend(loc=0)
plt.subplot(236)
plt.plot(X.cpu().numpy(),y.cpu().numpy(),label="real")
plt.plot(X.cpu().numpy(),y_pred[5].detach().cpu().numpy(),label="model_100")
plt.legend(loc=0)
plt.show()
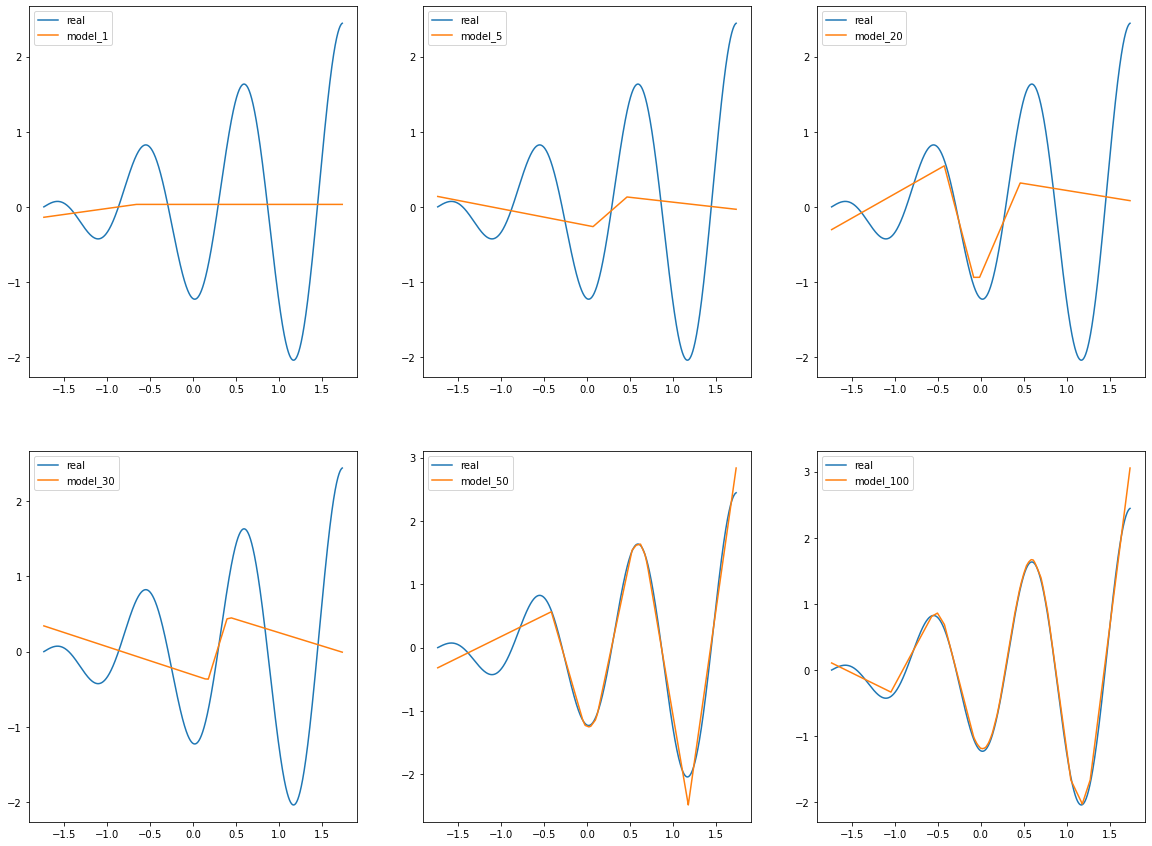